

It is important to mention here that when you use PCA for machine learning, the test data should be transformed using the loading vectors found from the training data otherwise your model will have difficulty generalizing to unseen data. In machine learning, on the other hand, by using the PCs, we will work with less number of columns and this can significantly reduce computational cost and help in reducing over-fitting. In data explorations, we can visualize the PCs and get a better understanding of processes using those PCs. In such scenarios where we have many columns and the first few PCs account for most of the variability in the original data (such as 95% of the variance), we can use the first few PCs for data explorations and for machine learning.
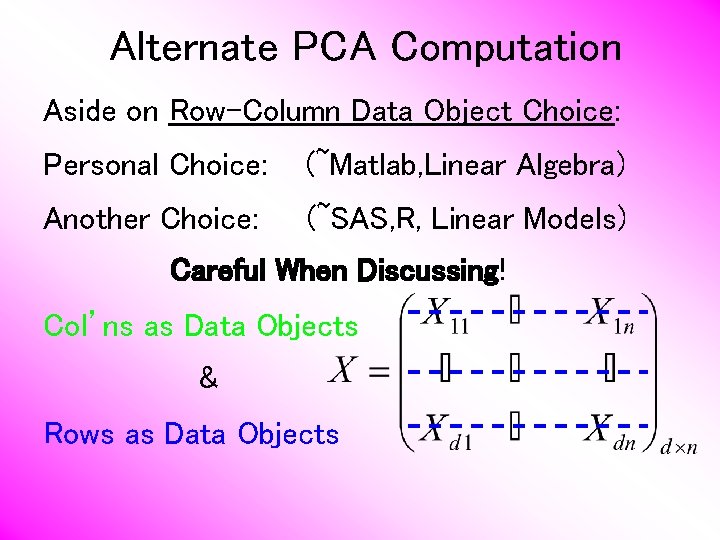
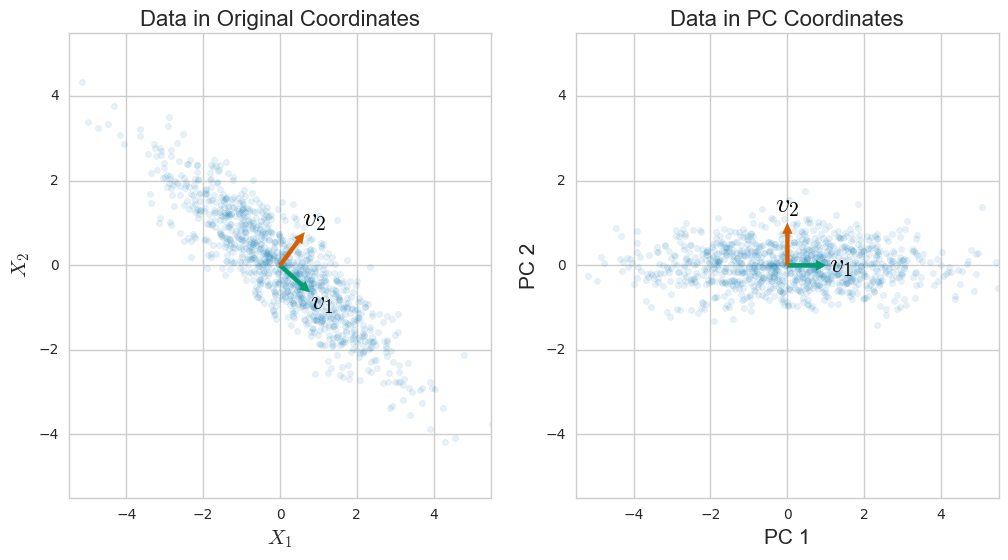
So, we see that the first PC explains almost all the variance (92.4616%) while the fourth PC explains only 0.5183%. The PCs are ranked based on the variance they explain: the first PC explains the highest variance, followed by the second PC and so on. The variance explained by each PC is shown below.
